Industry News
Top 5 Open Source AI Solutions for Image Processing
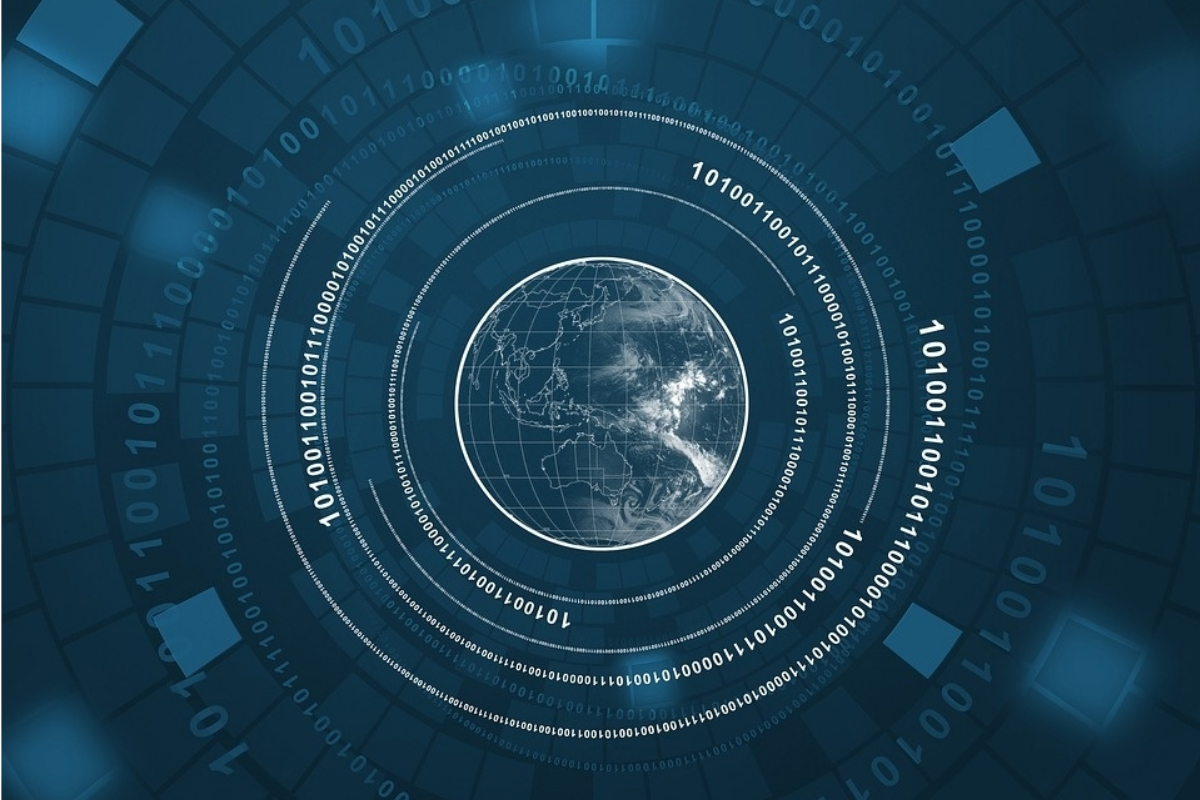
A few decades or even years ago, self-driving cars and computers with a human-like vision were just a figment of the fantasy writer’s imagination. But today, Artificial intelligence (AI) technologies allow cars to drive safely across busy streets and computers to interpret pictures almost like humans do.
Developers have made impressive progress on how to implement AI on image processing tasks. Furthermore, image processing solutions with AI are widely used in various fields, from medicine to law enforcement to cybersecurity and retail.
AI and machine learning make it possible for the machines to inherit new functionalities through the process of learning. Similarly to small kids, machines can gain the ability to acquire and understand visual information by processing massive amounts of images. To process so much data faster without compromising the final result, developers use image processing models based on machine learning and deep learning algorithms.
In particular, image processing and computer vision solutions use deep learning for accomplishing such complex tasks as:
- Image classification
- Object recognition
- Object tracking
- Image generation
- Image retrieval
To implement some computer vision functionalities into your product, you’ll need a large set of tools and resources: image datasets, libraries with code samples, frameworks for creating and training deep learning models. Fortunately, there are a lot of open-course services that you can use to make machine learning less of a mystery and ease the development of your own AI-based image processing solution.
Below, we take a closer look at the five best open source image processing solutions that you can apply to your projects:
- OpenCV
- TensorFlow
- Keras
- Caffe
- Google Colab
Let’s get started!
OpenCV
Open Source Computer Vision Library (OpenCV) is one of the most popular open source services for image processing. It’s a free computer vision library that you can use to perform various image processing tasks:
- Image acquisition
- Image compression and decompression
- Image enhancement and restoration
- Image denoising
- Image segmentation
- Data extraction, and more
The library includes numerous algorithms and functions as well as special modules aimed at image processing tasks. Starting from the library version 3.1, there’s also a deep learning module for building and training deep learning models.
With this module, you can use OpenCV to extract features from processed images, and then apply a machine learning model using one of the supported machine learning frameworks.
As of today, OpenCV supports a number of popular frameworks, including TensorFlow, PyTorch, and Caffe. The list of supported types of neural networks includes convolutional, deconvolutional, and recurrent neural networks.
The library is written in C++ and supports C++, Java, Python, and MATLAB interfaces.
TensorFlow
TensorFlow is an open-source machine learning framework created by Google. Initially, the project was started for research purposes of the Google Brain team. Today, however, TensorFlow is widely used by both small startups and large companies such as Dropbox, Intel, and Twitter.
You can use TensorFlow to process different types of data, but in relation to image processing, this framework works best for:
- Image classification
- Image recognition
- Image segmentation
- Image to image translation (pix2pix)
TensorFlow includes a set of libraries for creating and training custom deep learning models and neural networks. The framework supports Jupyter notebooks and provides a style guide with recommendations on writing readable, consistent code.
TensorFlow supports several popular programming languages, including C++, Python, Java, Rust, and Go. However, you can install third-party bindings for other languages, such as Ruby, Scala, or PHP.
Keras
Keras is an open-source Python library for creating deep learning models. It’s a great solution for those who only begin to use machine learning algorithms in their projects as it simplifies the creation of a deep learning model from scratch. Keras is easy to manage and it is suitable for fast and simple prototyping of different types of neural networks.
The library was built on TensorFlow and is currently fully integrated into the framework. This means that you can write your deep learning model in Keras, as it has a much more user-friendly interface, and then easily implement a specific functionality or feature from TensorFlow in this model.
Keras can also be deployed on top of other popular AI frameworks such as Microsoft Cognitive Toolkit and Theano.
Caffe
Convolutional Architecture for Fast Feature Embedding (Caffe) is an open-source framework that can also be used for creating and training popular types of deep learning architectures. You can use Caffe to accomplish such tasks as image classification, segmentation, and recognition.
Caffe is written in C++ but it also has a Python interface. The framework supports both CPU- and GPU-based accelerated libraries such as NVIDIA cuDNN and Intel MKL. The framework also has a special database, Caffe Model Zoo, containing a set of pre-trained deep learning models. As of today, it offers four BAIR-trained models:
- BAIR Reference CaffeNet
- BAIR Reference R-CNN ILSVRC-2013
- BAIR AlexNet
- BAIR GoogLeNet
Model Zoo also includes a number of community models trained by other Caffe users. As for the types of supported neural networks, Caffe works best with convolutional neural networks (CNN) and feedforward networks. At the same time, it’s not the best choice for training recurrent neural networks.
In 2017, Facebook launched Caffe2, an open-source framework for training and deploying deep learning models. And in 2018, Caffe2 was integrated with another popular AI framework, PyTorch.
Caffe2 comes with C++ and Python APIs and supports all popular platforms.
Google Colaboratory (Colab)
Google Colaboratory, or simply Colab, is one of the top image processing services. While it’s rather a cloud service than a framework, you can still use Colab for building custom deep learning applications from scratch. With the help of Colab, you can perform such image processing tasks as image classification, segmentation, and object detection.
Google Colab eases the use of other popular AI-based tools such as OpenCV, TensorFlow, and Keras. The service uses Jupyter Notebooks, helping developers to share their knowledge, tips, and best practices on building AI-based applications. Plus, in contrast to other similar services, Colab offers free usage of both CPU- and GPU-based acceleration.
Conclusion
Machine learning models and algorithms help developers implement specific image processing functionalities into their products both quickly and easily. However, building a custom machine learning model or neural network requires lots of resources and a high level of technology expertise. With the help of the listed open-source tools, libraries, and frameworks, you can simplify the process of leveraging Artificial Intelligence technologies to your benefit.
This article is a contribution from Marcell Gogan.  Marcell is a specialist within digital security solutions, business design and development, virtualization and cloud computing, R&D projects, establishment and management of software research direction – working with Ekran System. He also loves writing about data management and cybersecurity.Â
-
Asia7 days ago
Tencent Cloud Recognised as a Leader in Omdia Market Radar for Games in Asia & Oceania, Solidifying its Position as a Global Gaming Industry Innovator
-
Latest News7 days ago
Week 9/2025 slot games releases
-
Interviews7 days ago
2025 Sports Betting Roadmap: Exclusive Insights from SOFTSWISS
-
Asia6 days ago
S8UL Esports Unveils Power-Packed Call of Duty: Mobile Roster, sets its sights on national and international CODM glory
-
Latest News7 days ago
The Countries with the Most Interest in Gambling
-
Latest News4 days ago
2025’s Best Online Casinos Europe: Top 10 European Casino Sites, Rated by Experts
-
Latest News7 days ago
Playson cranks up the heat in 3 Chillies and Joker: Hold and Win
-
Industry News7 days ago
Amusnet CCO Irina Rusimova Unveils 2025 Business Strategy and Vision